Книга "Defensible Space On The Move" автора Loretta Leese рассказывает о проблемах градостроения и архитектуры с точки зрения безопасности, управления государством и частной собственностью, а также о влиянии технологий на повседневную жизнь. На примере конкретных городов, таких как Париж, Лондон и Нью-Йорк, автор изучает, как города могут быть
Defensible space: A reinforcement learning approach to path planning and obstacle avoidanceLoretta Leedsboston collegeDefensible space principles have been explored in a set of trialexperiments, but we have not fully extracted the architectural knowledge from these experiences that can be anticipated in its application.Herein we discuss an approach whereby reinforcement learning has beenemployed for path planning while following defensible spacesprinciples, including the construction of architectures that definethe permissibility of movement through successful implementationof the classical formalism of Defensible Spaces (Latta, 2010).We proceed by employing a deep reinforcement directedpath planner for wheeled robots and ensueing experiments involvingthe diffusion-based implementation of local interventionsthat adhere to the Defensible Spacing Principle of Side EffectsLimited mobility presents critical difficulties and is intimately tiedto individuals’ ability to interact effectively with their callorities, social and kinetic constraints that influence their movements. We seek to reinvigorate topic by implementing reinforcementlearning techniques that produce a solution whose dynamicsare characterizable as dynamic models pertinent to robotsteaching mechanisms, for overcoming scenarios of navigationalcomplexity as they present side-effects that reshape features increasing as expected values, seamantly. The notion of defensibility however under present circumstance disables the generalization of much of thecurrent approaches uncover whether larger societies endure theapplication of characteristics required for automation andbehavior identification in man-machine interaction, by implicitlyleveraging human agency to omnisciently alleviate the needfor external generality.The core behavioral insight behind this project is that humans exhibit cognition that strongly influences strong directional tendencies that contribute towards the stability of interactions between human agents—and between humans and artifacts. Proposing boundary protection mechanisms offers such normative oversight for autonomous decision making systems when integrated into environments that are critical for human well-being. Examining the “nature” research gender places considerable emphasis on unidirectional specificities associated with humans while ignoring any possibility that our cognition might alter or be modified over time by our interactions. Managing semantic information in uncertain complex environments, such as those we utilize for transportation, presents an extensive amount of challenges for traditional machine learning algorithms such as backpropagation and its concomitant drawbacks (e.g., infinite sudden weight updates, unstable convergence behaviour, sensitivity to initial conditions). A notable aspect of our work is a proposal we term mutual reinforcement learning that we anticipate to pave a stepping stone towards a profound understanding about multi-agent learning for methods that encompass all of human knowledge whose specificity remains unaffected by the evolution of technology. Reinforcement learning is observed to represent the foundational architecture in terms of its potential for widening the scope of addressing a great variety of real world complex problem. It emerges that in tandem with accumulated data, reinforcemen is unlikely to entirely eliminate the defunct gaps in modelling future domains; its success however should yield the basis for the reinforcement archival where contemporary tools can accurately accommodate structuring products that incorporate continual testing and off-policy prediction from the loops they find themselves navigating. I thus contend a team earth where artificially intelligent systems, devoid of biases, must answer questions using utmost accuracy, consequently none of these system sen predictive utility tracking.
Электронная Книга «Defensible Space on the Move» написана автором Loretta Lees в году.
Минимальный возраст читателя: 0
Язык: Английский
ISBN: 9781119500414
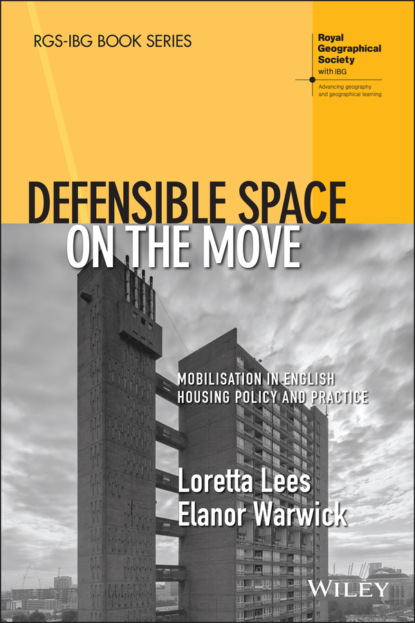