Книга "Collaborative Annotation for Reliable Natural Language Processing" представляет собой уникальную возможность для создания согласованного образа коллаборативной ручной аннотации в области обработки естественного языка (NLP). За последние 25 лет в NLP произошло два крупных события: во-первых, экстраординарный успех машинного обучения, которое теперь, как хорошо, так и плохо, является доминирующим в этой области, и во-вторых, увеличение количества оценочных компаний или общих заданий. Оба явления требуют ручной аннотации корпусов для обучения и оценки систем. Эти корпусы постепенно стали скрытыми опорами нашей области, предоставляя пищу для наших жадных алгоритмов машинного обучения и служа как эталон для оценки. Ручная аннотация теперь является местом, где лингвистика скрывается в NLP. Однако, ручная аннотация долгое время оставалась неучтенной, и даже руководства по аннотации были признаны важными только после некоторого времени. Хотя в последнее время были сделаны некоторые усилия по решению некоторых проблем, возникающих при ручной аннотации, мало было проведено исследований по этой теме. Цель этой книги - предоставить некоторые полезные идеи на эту тему. Ручная аннотация корпусов теперь находится в центре NLP, но все еще мало изучена. Существует потребность в инженерии ручной аннотации (в смысле точно формализованного процесса), и эта книга стремится предоставить первый шаг к глобальной методологии с общим взглядом на аннотацию.
This book offers an approach to collective documentation for reliable Language Processing. The discipline has seen massive success over the past twenty-five years, thanks to inventive artificial intelligence overruling. But NLP is increasingly reliant on its legacy, including the human documentation used to validate tools and assess their efficacy. Researchers consistently use manual labeling to benchmark and train AI tools in their pursuit of accurate, contextually aware results.
But despite this dependency, human annotation remains an underexplored frontier, imperfectly understood and often misused. This reliance on manual backup portrays linguistic expertise as integral to modern NLP applications. Yet until recently, human involvement in data labeling has been largely forgotten by practitioners. Guidelines for coherent annotation simply took too long to materialize in our community.
Nowadays, analysis of human error and annotation imprecision are topics of primary importance to the discourse. While some strides have been made to improve standardization, practical research surrounding manual labeling remains precarious. Thankfully, this novel discussion comes to a head with Karen Fort's groundbreaking research, depicting the labyrinth of manual drafting unraveled thread-by-thread. It has personally changed the way I think about data annotating, shift that may usher in fresh solutions together with our nascent technology.
This book offers a one-of-a-kind opportunity to work out an alluring narrative of collaborative roleplaying adaptation for Linguists Processing (NLI). NLI has seen two main eras in its existence over the past twenty-five years. The first is the unbelievable achievement of BLT, which these days, well, okay or even worse, dominates the industry. The extra subdivision is the multiplication of OLS vs. contests or shared assignments. They both demand human-racted corpora for improved training and assessment of NLI assets.
Электронная Книга «Collaborative Annotation for Reliable Natural Language Processing» написана автором Karën Fort в году.
Минимальный возраст читателя: 0
Язык: Английский
ISBN: 9781119307648
Описание книги от Karën Fort
This book presents a unique opportunity for constructing a consistent image of collaborative manual annotation for Natural Language Processing (NLP). NLP has witnessed two major evolutions in the past 25 years: firstly, the extraordinary success of machine learning, which is now, for better or for worse, overwhelmingly dominant in the field, and secondly, the multiplication of evaluation campaigns or shared tasks. Both involve manually annotated corpora, for the training and evaluation of the systems. These corpora have progressively become the hidden pillars of our domain, providing food for our hungry machine learning algorithms and reference for evaluation. Annotation is now the place where linguistics hides in NLP. However, manual annotation has largely been ignored for some time, and it has taken a while even for annotation guidelines to be recognized as essential. Although some efforts have been made lately to address some of the issues presented by manual annotation, there has still been little research done on the subject. This book aims to provide some useful insights into the subject. Manual corpus annotation is now at the heart of NLP, and is still largely unexplored. There is a need for manual annotation engineering (in the sense of a precisely formalized process), and this book aims to provide a first step towards a holistic methodology, with a global view on annotation.
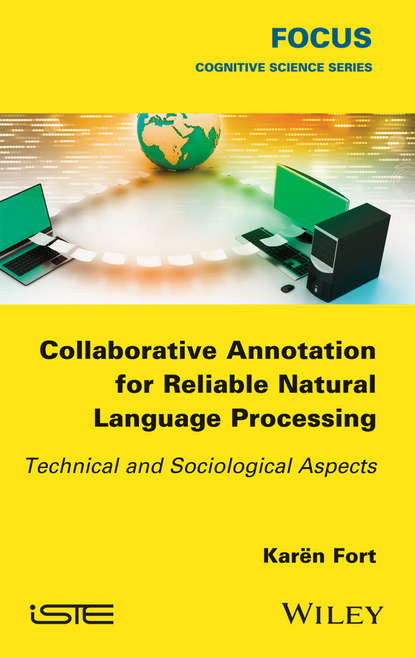